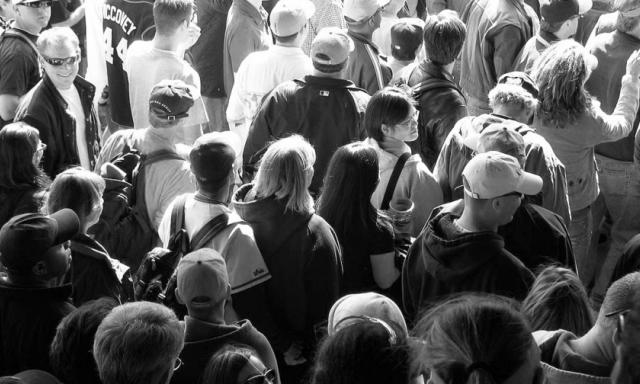
Too Many Expert Opinions in the Bunch? Trim Them Down
You may follow the predictions of experts in your industry in order to strategize for your company’s future, but is your approach effective? Our research series focusing on harnessing the wisdom of crowds examines methods of averaging expert forecasts and determining whether all experts are equal.
To improve the value gained from collectives of professional opinions, look to sizable crowds, use your best judgment about which experts to choose based on historical data and request diversity in the range of forecasts you will consider. However, there are some pitfalls to outmaneuver.
Trimming the Bunch
Along with fellow researcher Victor Richmond Jose of Georgetown University, we propose a new way to combine individual probability forecasts in the paper “Trimmed Opinion Pools and the Crowd’s Calibration Problem.”
While diversity in the opinion pools can help the average point forecast, it could hurt the probabilities forecast. Popular linear prediction pools can lean toward underconfidence. To get a more accurate picture of the likelihood of an event, trim the pool.
Two Ways to Trim
Exterior trimming removes the forecasts with low and high averages before calculating the pool’s average. Alternatively, you could consider trimming away, at every point, forecasts with low and high cumulative distribution function (CDF) values. Exterior trimming decreases the pool’s variance and improves its calibration. Interior trimming takes away forecasts with moderate means, or CDF values. This reduces overconfidence in an overconfident pool that is not diverse.
Quantiles or Probabilities?
A second paper, written with Duke University Fuqua School of Business Professor Robert L. Winkler, discusses the effectiveness of averaging quantiles — the points taken at intervals from the CDF values — over probabilities to aggregate expert opinions. In “Is It Better to Average Probabilities or Quantiles?” we found that averaging quantiles is a viable alternative to averaging probabilities and at times the preferred method. This research was published in Management Science.
When Are Quantiles Best?
The conditions under which averaging quantiles are best depend upon the agreement of experts on matters of location of the average, underconfidence or overconfidence of the predictions, and sharpness and shape.
- When experts disagree on the location of the mean, the average quantile forecast will tend to be more overconfident (or less underconfident) than the average probability forecast.
- In cases where there is more location disagreement and the average probability forecast is underconfident, the average quantile forecast will tend to score better because its increased sharpness leads to better calibration.
- When there is location agreement and the average probability forecast is overconfident, the more overconfident average quantile forecast may still score better. Our first two results involving shape concern the case of location agreement.
Why This Examination?
Forecasting allows firms to learn about possible outcomes of their decisions and helps them plan accordingly. Knowing the best way to elicit expert opinions and which combinations of opinions to use under the right circumstances helps decision-makers proceed with the best knowledge and predictions available.
Also, when organizations produce forecasts they need to understand the best ways to communicate their opinions.
For example, several organizations, such as The Wall Street Journal or the Federal Reserve Bank of Philadelphia collect and announce macroeconomic forecasts. These organizations need to figure out how to best collect forecasts from multiple experts and how to present them to the world.
In fact, the case Surveying Professional Forecasters explores the question of whether it’s best to “chase” a single expert’s opinion, or rely on the wisdom of crowds. The case presents examples of when individual experts might seem useful and when averaging the opinions of a crowd’s proves most useful.
The tension is that one entity relies on a single expert and even celebrates that expert when their predictions prove accurate. However, time shows that the single expert is not always right from year to year.
Source: (case data)
The lesson? Most often, the average of the crowd’s opinion is consistently accurate over time, and firms are often better off relying on the opinion average of the crowd.
Forecasting and making use of subjective probabilities are fundamental inputs in decisions. Decision makers often need to identify and analyze predictions, in situations in which everyone has a slightly different view of the future.
Professors Yael Grushka-Cockayne and Casey Lichtendahl co-authored the case Surveying Professional Forecasters (Darden Business Publishing, June 2013) to provide a basis for classroom discussion.